AI Engineering/TensorFlow
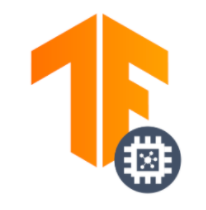
TFLite 뽀개기 (3) - Quantization
1. Quantization 내용은 python, Tensorflow-gpu 2.x, keras model 에 한정되어 있음을 알려드립니다. 이전 글에서 TFLite model로 Inference까지 해봤습니다. 이번에는 TFLite model을 경량화 시키는 방법을 알려드릴게요. 경량화 방법은 TFLite에서 제공하는 Quantization이며 경량화의 효과는 다음과 같습니다. Smaller storage size 모델 사이즈를 줄여 user의 device에 적은 storage을 occupy함. Less memory usage 작은 모델로 만들어 RAM memory를 적게 occupy함. Reducing latency 좀 더 빠르게 inference할 수 있게 함. 하지만 마냥 장점만 가져갈 수는 없죠..
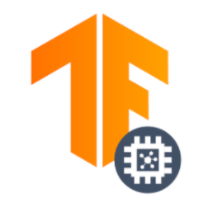
TFLite 뽀개기 (2) - TFLite Inference
1. TFLite Inference 내용은 python, Tensorflow-gpu 2.x, keras model 에 한정되어 있음을 알려드립니다. 이전 글로부터 만들어진 TFLite model로 이제 Inference을 해볼려고 합니다. 그 전에, Inference를 어디서 할 수 있는 지 알아야겠죠? Android Java 또는 C++ API를 사용하여 Inference iOS Swift 또는 Objective-C를 사용하여 Inference Linux C++ 또는 Python으로 제공되는 TFLite API를 사용하여 Inference 이번 글에서는 Linux부터 해볼게요.. 다시 돌아와서 Inference, 즉 prediction를 위해서는 TFLite API인 Interpreter을 주축으로 다..
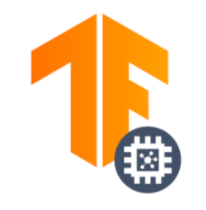
TFLite 뽀개기 (1) - TFLite 기본 이해 및 사용하기
1. TFLite 란? 해당 글은 python, Tensorflow-gpu 2.x, keras model 에 한정되어 있음을 알려드립니다. Tensorflow Lite (TFLite)는 mobile, embedding, IoT device에서 모델을 사용할 수 있도록 Tensorflow(keras) 모델을 변환해주는 tool입니다. 1.1 Tensorflow Lite convert 본격적으로 Keras model을 TFLite model을 변경하는 tool인 Tensorflow Lite convert 소개할게요. converter=tf.lite.TFLiteConverter.from_keras_model('your keras model') 위의 함수가 TFLite로 만드는 준비 compile과정이라고 생각..
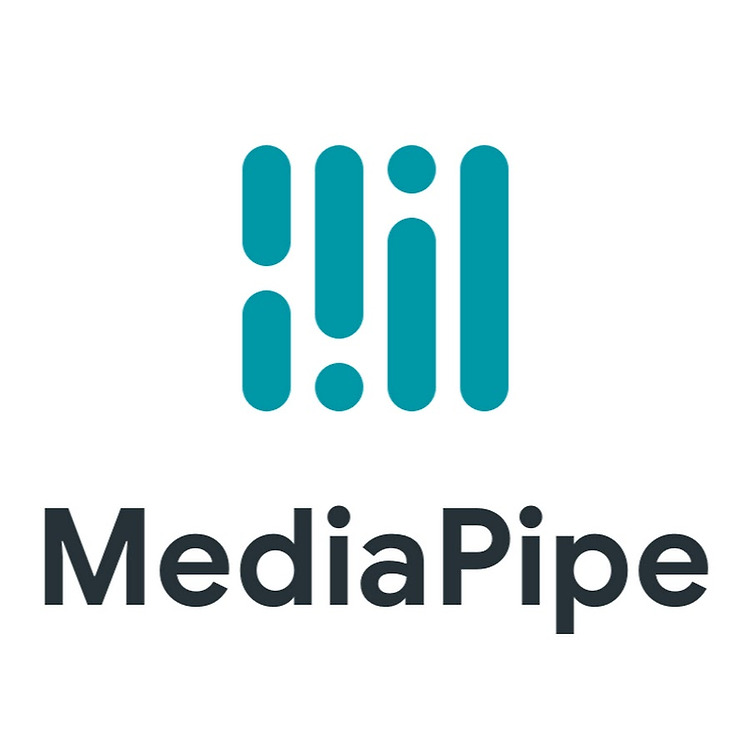
Mediapipe (1) - Mediapipe 이해 및 Object detection 예제
1. Mediapipe 란?? Mediapipe는 임의의 sensory data (e.g. images, video streams)에 대해 추론(inference)를 수행할 수 있도록하는 pipeline 구축을 위한 framework입니다. Mediapipe을 통해 Deep Learning(DL) model의 추론 pipeline을 module단위의 여러 요소들로 구성된 graph로 built하게 됩니다. 그래서 mediapipe는 mobile device나 IoT에 최적화된 deployment flow를 제공하게 되므로 on-device에 DL modeld을 deploy하고 싶으면 쓰면 좋을것 같네요. 1.1 Mediapipe 왜 써야하는가?? End-to-End acceleration ML model..