Model Compression
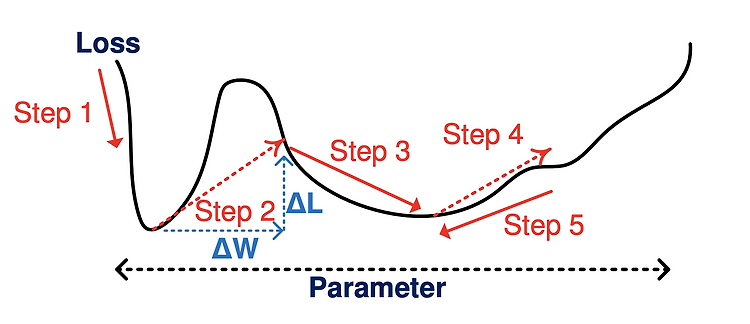
Learning Low-Rank Approximation for CNNs
1. Introduction Filter Decomposition (FD): Decomposing a weight tensor into multiple tensors to be multiplied in a consecutive manner and then getting a compressed model. 1.1 Motivation Well-known low-rank approximation (i.e. FD) methods, such as Tucker or CP decomposition, result in degraded model accuracy because decomposed layers hinder training convergence. 1.2 Goal To tackle this problem, t..
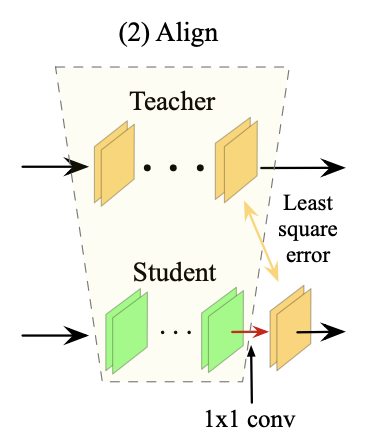
Few Sample Knowledge Distillation for Efficient Network Compression
1. Introduction Knowledge distillation: Dealing with the problem of training a smaller model (Student) from a high capacity source model (Teacher) so as to retain most of its performance. 1.1 Motivation The compression methods such as pruning and filter decomposition require fine-tuning to recover performance. However, fine-tuning suffers from the requirement of a large training set and the time..
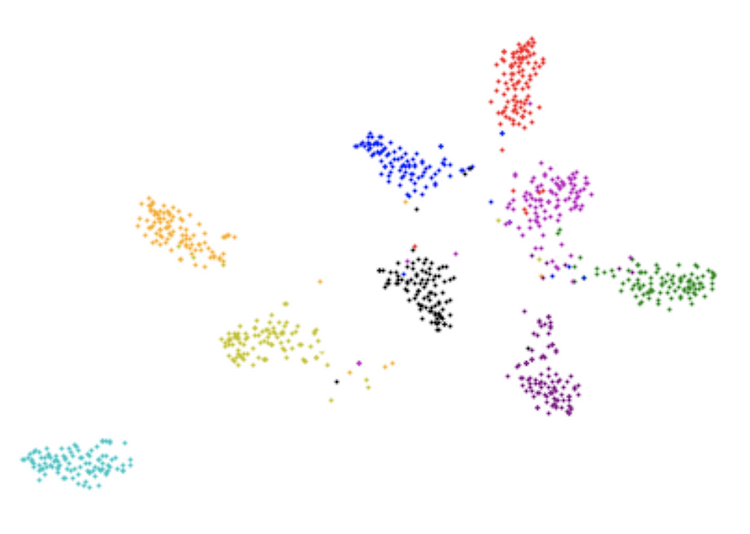
Knowledge Distillation via Softmax Regression Representation Learning
1. Introduction Knowledge Distillation: Dealing with the problem of training a smaller model (Student) from a high capacity source model (Teacher) so as to retain most of its performance. 1.1 Motivation The authors of this paper try to make the outputs of student network be similar to the outputs of the teacher network. Then, they have advocated for a method that optimizes not only the output of..
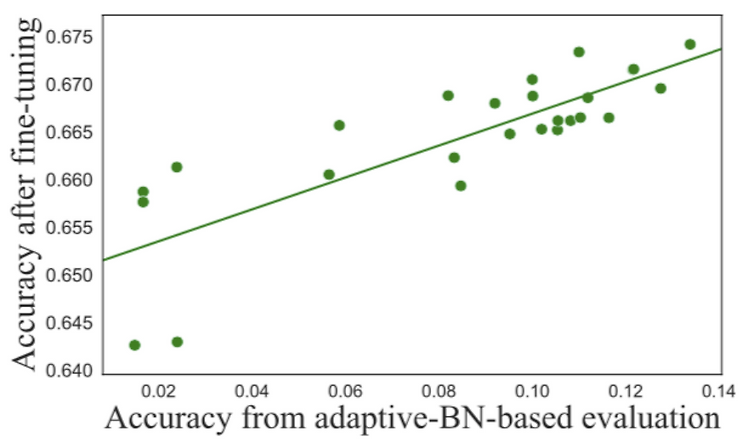
EagleEye: Fast Sub-net Evaluation for Efficient Neural Network Pruning
1. Introduction Pruning: Eliminating the computational redundant part of a trained DNN and then getting a smaller and more efficient pruned DNN. 1.1 Motivation The important thing to prune a trained DNN is to obtain the sub-net with the highest accuracy with reasonably small searching efforts. Existing methods to solve this problem mainly focus on an evaluation process. The evaluation process ai..
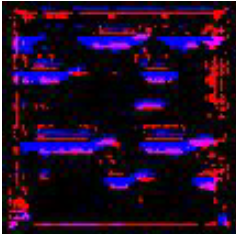
Data-Free Knowledge Amalgamation via Group-Stack Dual-GAN
1. Goal The goal is to perform Data-free Knowledge distillation. Knowledge distillation: Dealing with the problem of training a smaller model (Student) from a high capacity source model (Teacher) so as to retain most of its performance. As the word itself, We perform knowledge distillation when there is no original dataset on which the Teacher network has been trained. It is because, in real wor..
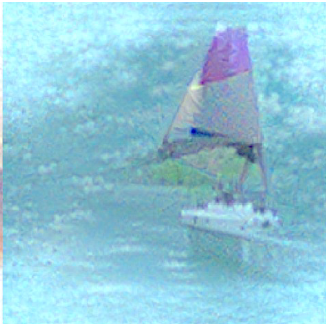
Dreaming to Distill Data-free Knowledge Transfer via DeepInversion
1. Goal The goal is to perform Data-free Knowledge distillation. Knowledge distillation: Dealing with the problem of training a smaller model (Student) from a high capacity source model (Teacher) so as to retain most of its performance. As the word itself, We perform knowledge distillation when there is no original dataset on which the Teacher network has been trained. It is because, in the real..