da2so
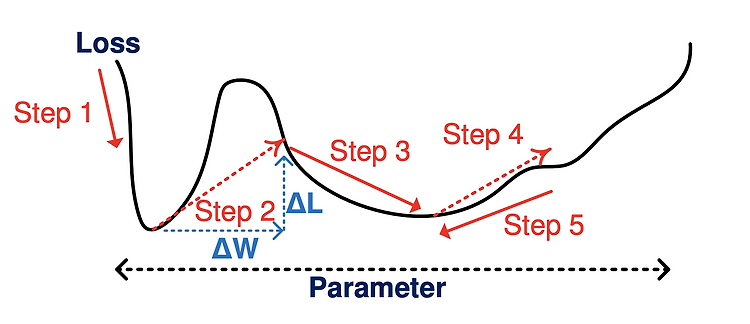
Learning Low-Rank Approximation for CNNs
1. Introduction Filter Decomposition (FD): Decomposing a weight tensor into multiple tensors to be multiplied in a consecutive manner and then getting a compressed model. 1.1 Motivation Well-known low-rank approximation (i.e. FD) methods, such as Tucker or CP decomposition, result in degraded model accuracy because decomposed layers hinder training convergence. 1.2 Goal To tackle this problem, t..
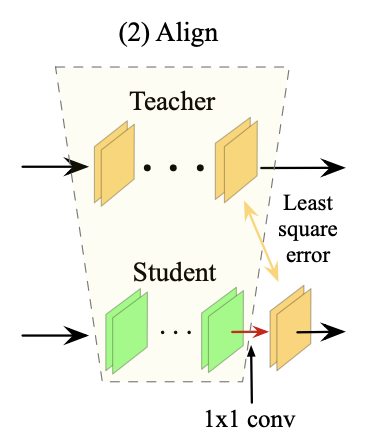
Few Sample Knowledge Distillation for Efficient Network Compression
1. Introduction Knowledge distillation: Dealing with the problem of training a smaller model (Student) from a high capacity source model (Teacher) so as to retain most of its performance. 1.1 Motivation The compression methods such as pruning and filter decomposition require fine-tuning to recover performance. However, fine-tuning suffers from the requirement of a large training set and the time..
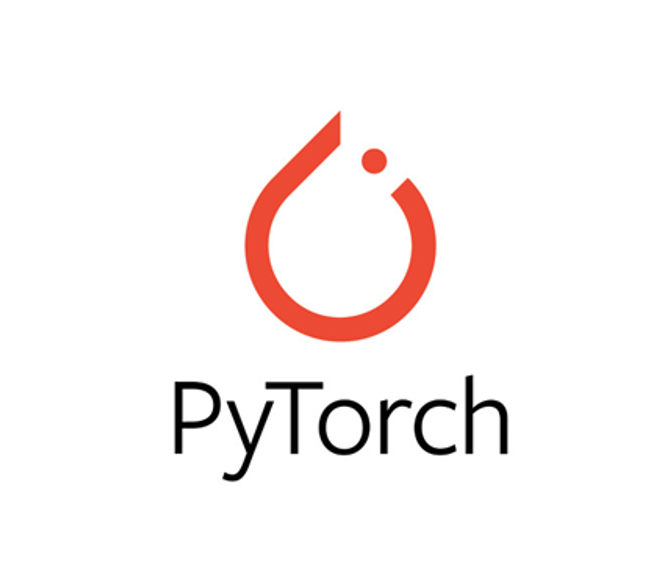
PyTorch MultiGPU (1) - Single-GPU vs Multi-GPU (DataParallel)
1. Introduction 모든 실험은 python 3.6, Pytorch 1.7.0 에서 진행되었음을 알려드립니다. 해당 글은 Pytorch에서 Single-GPU와 Multi-GPU의 차이를 이해하고 직접 실험해 볼 수 있는 환경을 제공하기 위함을 알려드립니다. 오늘 설명드릴 목차는 다음과 같습니다. Experiment setting Single-GPU vs Multi-GPU (DataParallel) 기본 이해 Single-GPU vs Multi-GPU (DataParallel) 결과 비교 Multi-GPU (DataParallel) 의 문제점 2. Experiment Setting Single-GPU와 Multi-GPU의 차이에 대해 글로 설명을 드릴거지만 직접 코드를 돌려보면서 이해하시는 게 ..
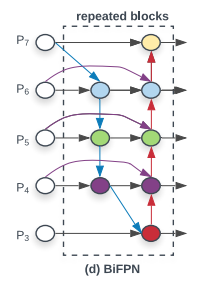
EfficientDet Scalable and Efficient Object Detection
1. Introduction 1.1 Motivation The existing methods for object detection mainly have two problems. (i) Most previous works have developed network structures for cross-scale feature fusion. However, they usually contribute to the fused output feature unequally. (ii) While previous works mainly rely on bigger backbone networks or larger input image sizes for higher accuracy, scaling up feature net..
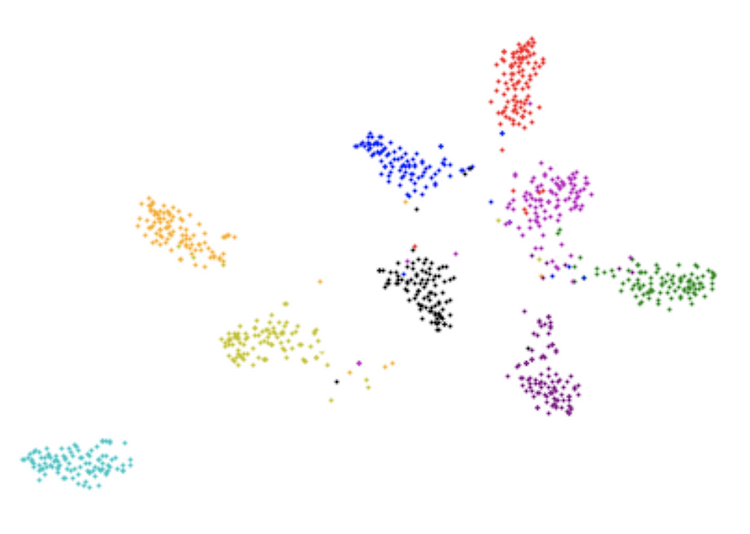
Knowledge Distillation via Softmax Regression Representation Learning
1. Introduction Knowledge Distillation: Dealing with the problem of training a smaller model (Student) from a high capacity source model (Teacher) so as to retain most of its performance. 1.1 Motivation The authors of this paper try to make the outputs of student network be similar to the outputs of the teacher network. Then, they have advocated for a method that optimizes not only the output of..
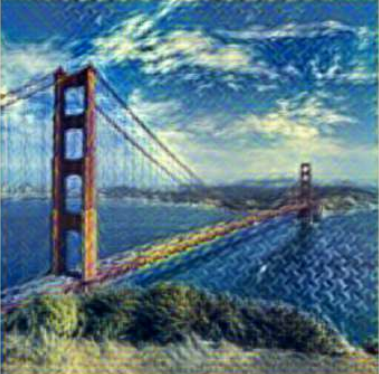
Adjustable Real-time Style Transfer
1. Introduction Style transfer: Synthesizing an image with content similar to a given image and style similar to another. 1.1 Motivation There are two main problems in style transfer on the existing methods. (i) The first weak point is that they generate only one stylization for a given content/style pair. (ii) One other issue of them is their high sensitivity to the hyper-parameters. 1.2 Goal T..
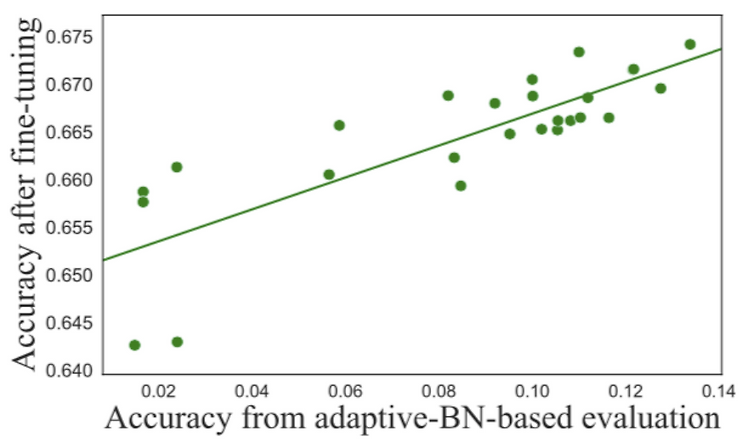
EagleEye: Fast Sub-net Evaluation for Efficient Neural Network Pruning
1. Introduction Pruning: Eliminating the computational redundant part of a trained DNN and then getting a smaller and more efficient pruned DNN. 1.1 Motivation The important thing to prune a trained DNN is to obtain the sub-net with the highest accuracy with reasonably small searching efforts. Existing methods to solve this problem mainly focus on an evaluation process. The evaluation process ai..