AI paper review
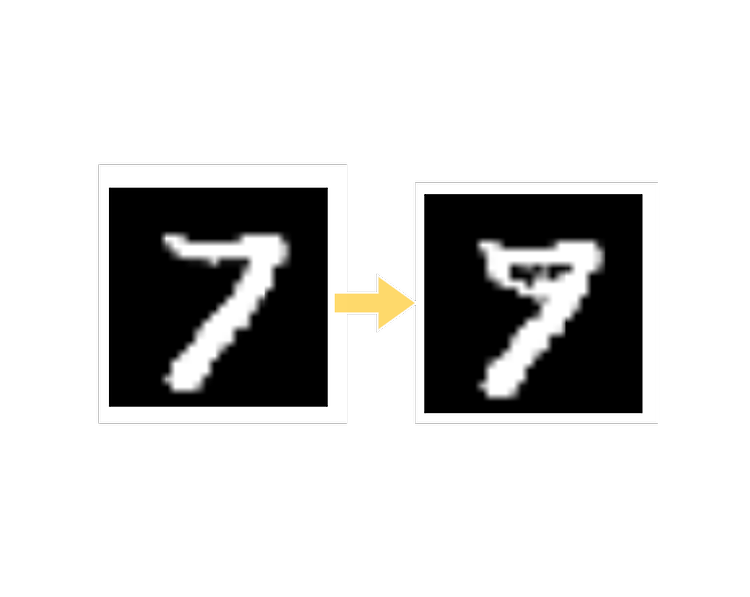
Counterfactual Explanation Based on Gradual Construction for Deep Networks
1. Counterfactual Explanation Counterfactual Explanation: Given input data that are classified as a class from a deep network, it is to perturb the subset of features in the input data such that the model is forced to predict the perturbed data as a target class. The Framework for counterfactual explanation is described in Fig 1. From perturbed data, we can interpret that the pre-trained model t..
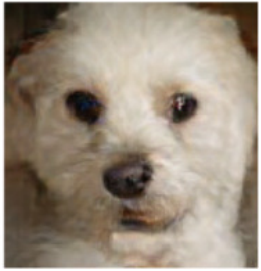
A Disentangling Invertible Interpretation Network for Explaining Latent Representations
1. Interpreting hidden representations 1.1 Invertible transformation of hidden representations Input image:
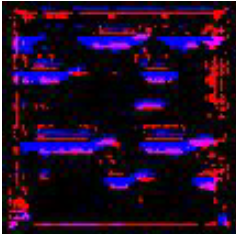
Data-Free Knowledge Amalgamation via Group-Stack Dual-GAN
1. Goal The goal is to perform Data-free Knowledge distillation. Knowledge distillation: Dealing with the problem of training a smaller model (Student) from a high capacity source model (Teacher) so as to retain most of its performance. As the word itself, We perform knowledge distillation when there is no original dataset on which the Teacher network has been trained. It is because, in real wor..
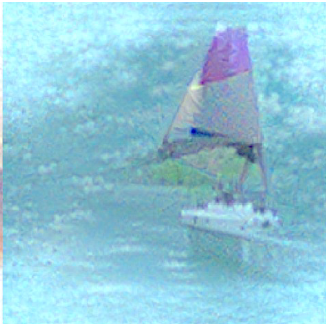
Dreaming to Distill Data-free Knowledge Transfer via DeepInversion
1. Goal The goal is to perform Data-free Knowledge distillation. Knowledge distillation: Dealing with the problem of training a smaller model (Student) from a high capacity source model (Teacher) so as to retain most of its performance. As the word itself, We perform knowledge distillation when there is no original dataset on which the Teacher network has been trained. It is because, in the real..
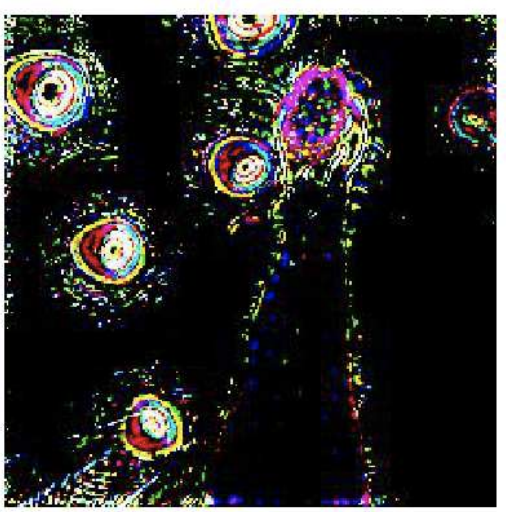
Interpretable And Fine-grained Visual Explanations For Convolutional Neural Networks
1. Goal In Interpretable And Fine-grained Visual Explanations For Convolutional Neural Networks, authors propose an optimization-based visual explanation method, which highlights the evidence in the input images for a specific prediction. 1.1 Sub-goal [A]: Defending against adversarial evidence (i.e. faulty evidence due to artifacts). [B]: Providing explanations which are both fine-grained and p..
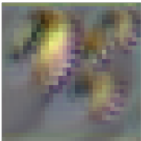
Zero-Shot Knowledge Transfer via Adversarial Belief Matching
1. Data-free Knowledge distillation Knowledge distillation: Dealing with the problem of training a smaller model (Student) from a high capacity source model (Teacher) so as to retain most of its performance. As the word itself, We perform knowledge distillation when there is no original dataset on which the Teacher network has been trained. It is because, in real-world, most datasets are proprie..